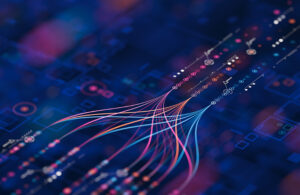
[Adobe Stock]
Do you think the AI boom is a bubble?
While the current surge in AI interest will likely cool down eventually, it would be wrong to assume that an AI winter is coming soon.
Consider that AVNet's latest survey of 1,200 electronics engineers found that eight out of 10 are actively involved in AI, with 42% already building AI-based products and another 40% incorporating AI into upcoming designs. And it's not just about products themselves – 95-96% of respondents see AI as “something” “extremely likely” to transform multiple aspects of the product design process.
Oh, and AI tools are hot too. Nearly two-thirds of respondents, 64%, used ChatGPT when searching for information during design and development. And Google Gemini, Microsoft Copilot and Meta AI were not far behind. Only 6% said they have not used such AI tools.
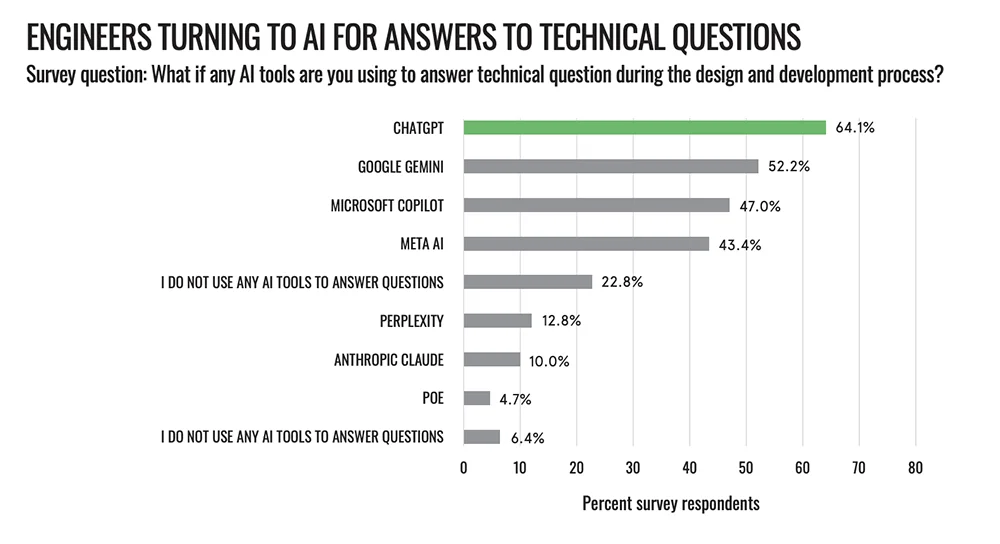
[From the ‘Embracing AI‘ Avnet survey]
Compare the situation with the Internet of Things, which experienced a similar hype cycle. While IoT's buzz eventually faded as it was integrated into broader technology, generative AI appears to be on a different path. In fact, data from the St. Louis Fed shows that early adoption of Genai has already surpassed initial PC and Internet uptake, hovering around 40% just a few years after its debut. While some surveys have found that hiring employees in 2024 appears cool, KPMG's survey in AI Quarterly Pulse found that 68% of executives would expect between $50 million and $250 million in the next 12 months Genai Invest – strong from 45% in early 2024.
Morgan Stanley analyst Brian Nowak has forecast that 2025 capex for the hyperscalers will exceed $300 billion – almost double 2023 levels. The economist noted that AI data center spending could eclipse $1.4 trillion by 2027. The technology spreads safely [of AI]“Said Alex Iuorio, SVP, Global Supplier Development at AVNET.
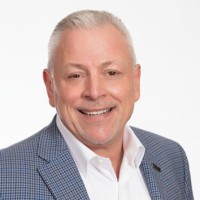
Alex Iuorio
As AI dynamics continue, IUORIO recognizes the cyclical nature of the technology. “It would be a bit silly to think it will take forever for these kinds of prices.” Still, he cautions against premature predictions of a slowdown, noting: “Predicting the slowdown when there doesn't seem to be any sign of it… that's an interesting position.”
Investors have conflicting views on the AI market. For one thing, Goldman Sachs said in September that AI stockers were not in a bubble. However, there are some potential warning signs. NVIDIA's price-to-sales ratio of around 30 and Palantir's nearly 68, according to recent valuations, recalls memories of the inflated valuations of the dot-com bubble. Concerns about diminishing returns from larger AI models and regulatory hurdles, particularly in the EU, further fuel these concerns. However, IUORIO sees continued demand growth, particularly as AI expands to industrial advantage. “If Ai gets it, if it gets to the rim, it will be exciting,” Iuorio said.
The rapid expansion and investment in AI technologies is also reminiscent of the rapid spread of IoT in previous years, leading to widespread security vulnerabilities that continue to plague networks today. Understandably, almost one in four respondents, 37.5%, see security and privacy as the biggest concern for AI projects, followed by data quality at 30.6%.
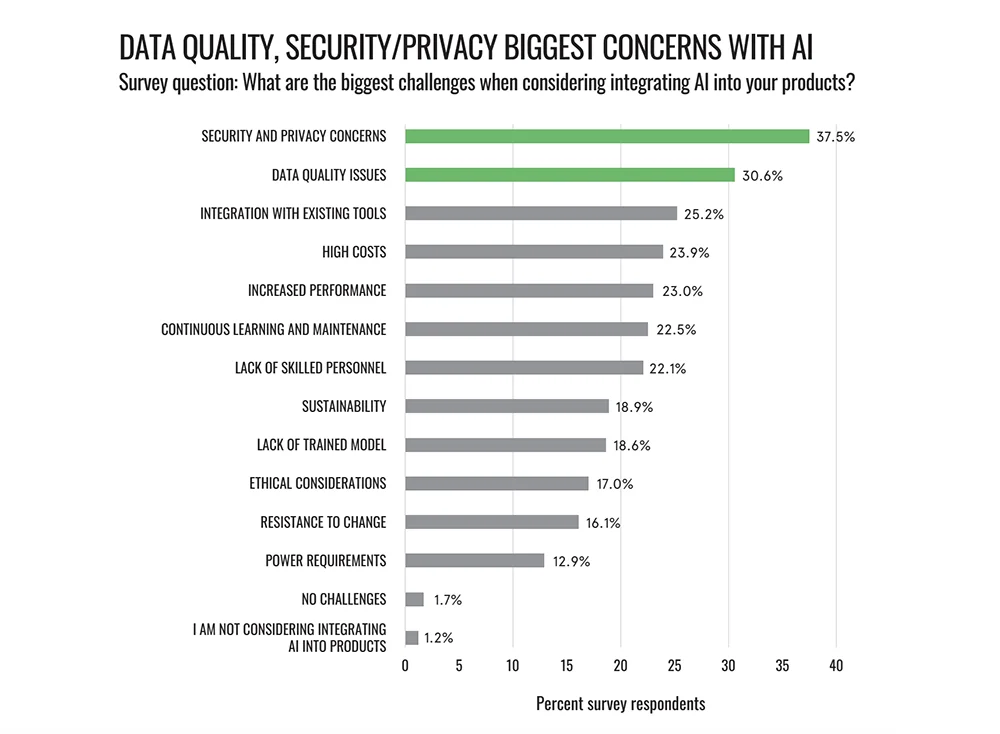
[From the ‘Embracing AI‘ Avnet survey]
The switch back to hardware
In the 80s, hardware design was about differentiation. …Today, hardware differentiation has been shifted to software. Now Ai turns it back.
For decades, the tech industry placed a premium on software-centric innovation. In 2011, Marc Andresen stated that “software is eating the world.” But in the 1980s, hardware was cool. In the early years of the decade, Chicago was a thriving center for coin-operated gaming. Major companies such as Midway (PAC-Man), Williams Gaming, Atari and Rock-Ola were all based there or had a large presence in the region, driving significant demand for electronic components.
“It was an incredible time to be in hardware,” Iuorio recalled. Engineers back then had the buildings out – sometimes literally in the parking lot – to show off new logic boards with no jumper, a feat that improved manufacturing and speed. The sheer excitement surrounding such design breakthroughs shows how much hardware matters. “You would package a case, a hard drive, a display and then the software into an ATM. But early on it was hardware that gave you an edge,” he said.
Organizations ranging from Finance Times to Deloitte agree that the pendulum is swinging back. Today's AI workloads – particularly in generative models – require significant computing power, specialized storage architectures, and advanced cooling and power management. As a result, hardware is once again the linchpin for differentiation.
Meanwhile, hyperscalers are buying GPUs in record quantities and snapping up advanced chipsets for data center AI training. In January 2025, OpenAI – with SoftBank, Oracle and MGX – plugged the Stargate project, a commitment of up to $500 billion over four years to build AI data centers in the US. Central Park in size). Previously, Elon Musk's XAI venture announced that the Colossus supercomputer with 100,000 Nvidia H100 GPUs in Memphis plans to scale to a million GPUs by 2027.
The gap between hyperscalers and industrial AI adoption
“The market is crazy when you're associated with AI and the chips that support it, but from an industrial market perspective it's not that exciting-yet.”
With consumer AI dominating the headlines – from hyperscalers taking on massive GPU clusters – many industry players have been slower to get on board. The reasons vary from cost constraints to complexities related to retrofitting legacy systems. However, AVNET's survey points to a growing wave of industrial applications. Almost half of respondents see AI as key to processing automation (42%), predictive maintenance (28%) or error detection (27%) – use cases that promise to increase efficiency without relying solely on public clouds. Meanwhile, industry giants from Honeywell to Siemens have significant AI initiatives and partnerships.
In the world of consumer tech, AI has gone from novelty to necessity in record time. But in industrial settings – factories, energy networks or even hospital equipment – adoption remains more conservative.
In addition to increased regulatory requirements, many industrial companies maintain equipment that can be decades old, making it difficult to integrate AI models and edge-ready hardware. IUORIO notes that it is “very timely” to conduct this survey and see how AI can apply to the design process. The interest in medical applications of AI is clearly to build drug discovery into surgical robotics.
New technology requires new skills
The AVNET report shows a broad distribution of required skills, with AI model optimization (16.5%), data analysis (16.2%) and problem solving (15.8%) being almost equally important. These capabilities are not randomly distributed, explains IUORIO, but represent “categorical” elements that will “successfully design and deploy AI technologies.”
Ultimately, while generative AI garners a lot of headlines, IUORIO sees a far deeper impact once these algorithms step out of the data center and into the real world. As he puts it, “When it gets to the edge, it gets exciting.”